A Robust Adversarial Immune-Inspired Learning System (RAILS)
TECHNOLOGY NUMBER: 2021-141
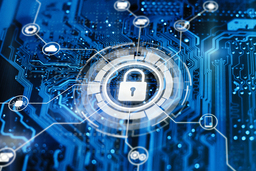
OVERVIEW
A new adversarial defense framework that is inspired by the immune system's powerful defense ability- Robustifies deep learning architectures and hardens Deep k-Nearest architectures against evasion attacks
- Delivers improvement in robustness without appreciable loss of accuracy as compared to applying DkNN alone
BACKGROUND
State of art in supervised learning, especially deep learning, has dramatically improved over the past decades. Many techniques are widely used as effective tools aiding human tasks, e.g., face recognition, object detection, natural language processing. However, despite effectiveness, deep learning techniques have all been demonstrated vulnerable to imperceptibly examples intentionally designed by evasion attack (i.e., adversarial attack). The vulnerability of deep neural networks (DNN) restricts its application scenarios and motivates researchers to develop various defense techniques.
INNOVATION
Researchers at the University of Michigan have developed Robust Adversarial Immune-Inspired Learning System (RAILS), a new adversarial defense framework that is inspired by the immune system's powerful defense ability. The researchers employ an adaptive immune system emulation, which emulates the immune system's defense mechanisms in order to robustify deep learning architectures and harden Deep k-Nearest (DkNN) architectures against evasion attacks. The life-long learning mechanism allows RAILS to evolve and defend against diverse attacks. When applied to adversarial image classification, the performance of the RAILS implementation delivers an additional 5.62% improvement in robustness without appreciable loss of accuracy as compared to applying DkNN alone.
PATENT APPLICATION
Number: Application number 63/123,684