Adaptive Input Estimation for Enhanced Tracking of Maneuvering Targets
TECHNOLOGY NUMBER: 6896
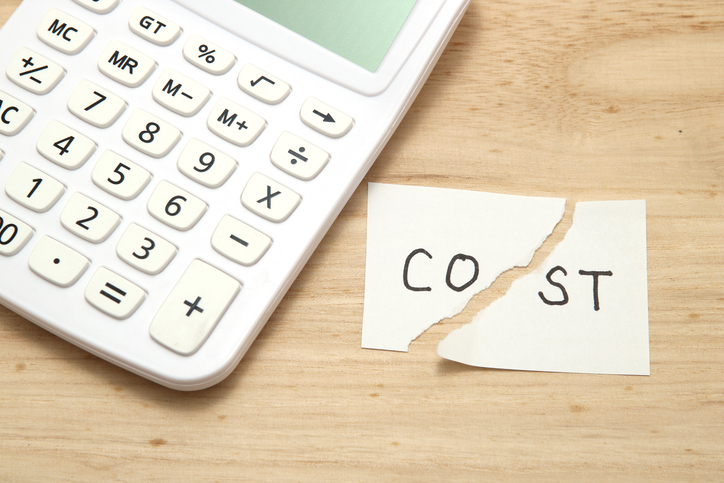
OVERVIEW
Novel adaptive state estimation without prior noise statistics knowledge
- Improves accuracy in dynamic and uncertain modeling scenarios
- Applicable in aerospace, defense systems, and robotics
BACKGROUND
State estimation has been fundamental in control theory for over half a century, with the Kalman filter being the most notable tool. This filter estimates the states of dynamic systems by weighing model predictions and real-time data, assuming the availability of knowledge concerning the system’s noise characteristics and dynamics. Nonetheless, real-world systems often come with uncertainties in noise statistics and dynamics, significantly degrading filter performance. Practitioners have tried to address these uncertainties using adaptive and robust methods, yet these innovations incorporate significant complexity and often require prior knowledge or assumptions that might not always hold true in varied operational conditions. Thus, a gap exists for a more flexible approach to state estimation that can handle unknown noise statistics and uncertain dynamics without relying on pre-existing statistical information.
INNOVATION
Researchers at the University of Michigan have developed Adaptive Retrospective Cost State Estimation (RCSE) algorithm that produces an innovative solution by circumventing the conventional dependency on noise statistic knowledge. Instead of traditional methods, it uses the innovations error to adaptively update the state estimates via retrospective cost optimization. With retrospective cost, RCSE refines the estimates in response to past performance and predictions, offering robust adaptability to uncertain dynamics and noise in systems. This unique approach simplifies the estimation process and enhances accuracy, even when substantial system uncertainties exist. The potential applications are broad, with significant utility in fields such as aerospace engineering for flight dynamics models, defense systems for tracking and surveillance, or any complex systems requiring precise state estimation without prior noise information.
ADDITIONAL INFORMATION
REFERENCES
A. Ansari and D. S. Bernstein, "Adaptive input estimation for nonminimum-phase discrete-time systems," 2016 IEEE 55th Conference on Decision and Control (CDC), Las Vegas, NV, USA, 2016, pp. 1159-1164, doi: 10.1109/CDC.2016.7798423