Automated Segmentation and Severity Analysis of Liver Parenchymal Disruption
Technology number: 2021-290
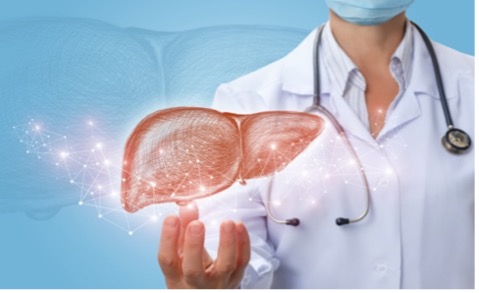
OVERVIEW
Automated quantitative assessment of liver trauma using deep learning
- Reduces observer variability and enhances trauma severity assessment
- Useful for trauma diagnostics, triage tools, volumetric injury monitoring
BACKGROUND
Liver trauma accounts for approximately 5% of all trauma admissions due to the liver's large size, anterior location, and fragile parenchyma. Early detection and appropriate treatment of liver injuries can significantly reduce morbidity and mortality. The gold standard for evaluating liver trauma is contrast-enhanced computed tomography (CT), with the American Association for Surgery Trauma (AAST) grading scale being the primary tool for assessment. However, this scale relies heavily on the subjective visual assessment of liver parenchyma disruption, leading to high intra- and inter-observer variability and potentially inaccurate quantifications. Thus, a need exists for an objective, automated approach to enhance liver trauma assessments.
INNOVATION
Researchers have created a fully automated image processing and deep learning framework to quantitatively assess liver trauma, addressing the limitations of subjective visual assessments. The framework utilizes deep convolutional neural networks (CNNs) to analyze three-dimensional contrast-enhanced CT scans, generating initial masks for both liver parenchyma and trauma-affected regions. Post-processing integrates domain knowledge to minimize false positives. The system accurately computes liver disruption involvement (LDI) by calculating the percentage of liver parenchyma disrupted by trauma. The model demonstrates high precision, recall, and Dice coefficients. Real-world applications include enhancing trauma diagnostics, serving as a triage tool, and monitoring the volumetric progression of liver injuries, thus guiding better management and treatment decisions in clinical practice.