Bayesian Training Guide
Technology Number: 2025-167
Technology No. 2025-167
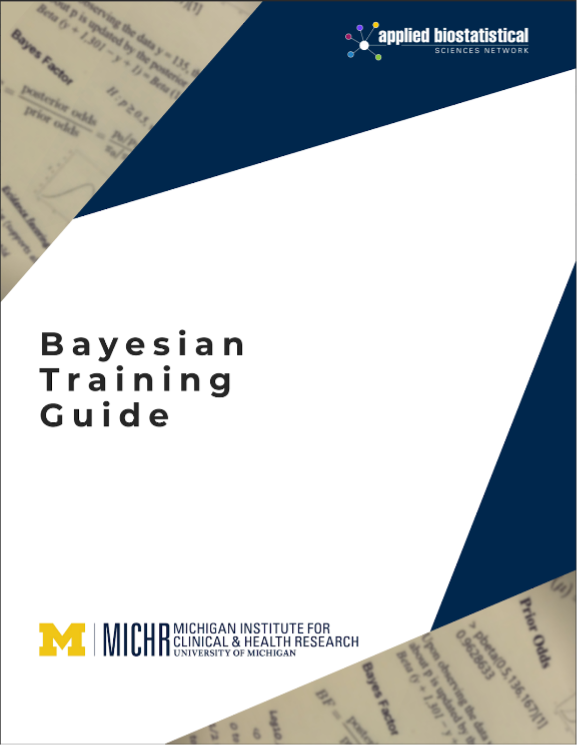
1
OVERVIEW
- Guide to implementing a Bayesian training program for research professionals within institutions.
- Freely available under Creative Commons CC BY-NC.
INNOVATION
The Bayesian Training Guide for biostatisticians was developed by the University of Michigan Applied Biostatististical Sciences (ABS) Network and Michigan Institute for Clinical & Health Research. In this guide, MICHR maps a template for providing high quality Bayesian training to replicate at other institutions. This guide outlines the need for Bayesian training and includes examples of learning outcomes, session topics, institutional supports, and coursework recommendations to build a solid Bayesian training program.
-
swap_vertical_circlemode_editInventor (4)Shokoufeh KhalatbariClare SansburnJordan HahnBrenda Eakin
-
swap_vertical_circlecloud_downloadSupporting documents (1)Product brochureBayesian Training Guide.pdfAdditional files may be available once you've completed the transaction for this product. If you've already done so, please log into your account and visit My account / Downloads section to view them.