Predicting Intensive Care Transfers and other Unforeseen Events (PICTURE)
Technology number: 2019-094
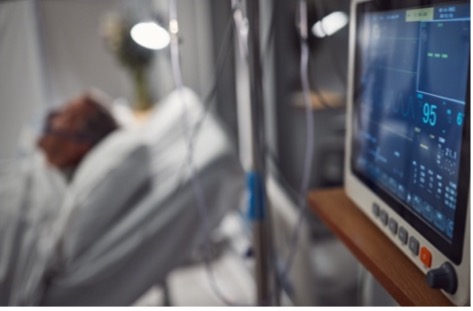
OVERVIEW
Machine learning algorithm for predicting ICU transfer and patient deterioration
- Provides accurate, explainable predictions using electronic health records
- Hospital patient monitoring, ICU transfer prediction, clinical decision support
BACKGROUND
Identifying patient deterioration in hospital wards is crucial but challenging. Early detection can reduce mortality by 3.5 times, shorten hospital stays by five days, and save significant costs per patient. Historically, clinicians have relied on rule-based scores like the Modified Early Warning Score (MEWS) and the Rothman Index, or statistical learning systems like eCART. While advanced, these systems still produce many false alarms and lack reliable prediction capabilities, often failing to aid clinicians in making confident decisions. There is a critical need for a more advanced approach that can accurately predict patient deterioration while providing actionable insights into the reasons behind each prediction.
INNOVATION
Researchers have developed a machine learning algorithm, PICTURE (Predicting Intensive Care Transfers and other Unforeseen Events), which utilizes electronic health record (EHR) data to predict ICU transfer or patient deterioration accurately. This gradient boosting tree algorithm leverages vital signs, lab results, and other patient indicators, transforming data into 8-hour intervals for analysis. PICTURE uniquely identifies the top contributing factors for each prediction using SHAP (SHapley Additive exPlanations), providing clinicians with clear insights. With a high area under the receiver operator characteristic curve (AUC) and superior performance in comparison to existing indices like eCART and Rothman, PICTURE offers a robust, explainable solution for patient monitoring. Real-world applications include enhanced hospital patient monitoring, predicting ICU transfers, and supporting clinical decision-making to improve patient outcomes.