Sequential Minimal Optimization Algorithm for Learning Using Partially Available Privileged Information
TECHNOLOGY NUMBER: 2019-068
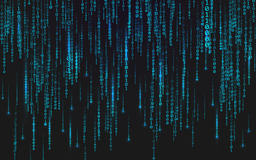
OVERVIEW
An enhanced support vector machine (SVM) that aids clinical decision support systems (CDSS)- Provides an ability learn partially available privileged information
- Addresses data that has gaps or is corrupted to provide high confidence predictions
BACKGROUND
Support vector machine (SVM) is a linear model for solving classification and regression problems. It is useful in the evaluation of applications like handwriting recognition, intrusion detection, face detection, email classification, gene classification, and in web pages. SVM may aid the progression from experience-based medicine to evidence-based practice relying on data analysis in settings of low evidential basis. This type of clinical decision support system (CDSS) provides a program module that helps medical professionals make decisions at the point of care.
INNOVATION
Researchers have developed a technique which enhances machine learning systems implemented while using privileged information configuration. The application combines the ability to improve functionality at testing phases using an advanced SVM approach which serves as a "gap filling" model. The supervised learning system can access, analyze, and use privileged information available for training data which is not available for test data. The supervised learning systems can also therefore learn where privileged information is known for all or part of the training data. The ability to learn partially available privileged information may combine a sequential minimal optimization (SMO) process to implement such learning. One clinical example of its usefulness would be the ability to diagnose acute respiratory distress syndrome (ARDS) by combining information that includes processing wavelet signal data from an electrocardiogram, combining cognitive and electronic health record data which evaluates the patient's physical status, and processing features of recent radiographic studies.
-
swap_vertical_circlemode_editInventor (7)Elyas SabetiJonathan GryakJoshua DrewsKayvan NajarianKevin WardMichael SjodingTheodore Iwashyna
-
swap_vertical_circlecloud_downloadSupporting documents (1)Product brochureSequential Minimal Optimization Algorithm for Learning Using Partially Available Privileged Information.pdf