VIME: Visual Interactive Model Explorer for Identifying Capabilities and Limitations of Machine Learning Models
Technology number: 2024-124
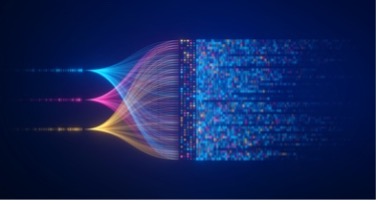
OVERVIEW
Explainability tool for sequential Machine Learning (ML) model decision analysis
- Enhances understanding of sequential ML decisions through interactive "what-if" scenarios
- Useful for high-stakes fields including healthcare, finance, and autonomous systems
BACKGROUND
Machine Learning (ML) models have become integral to various domains, from healthcare to finance, where their decisions can have significant consequences. One traditional challenge has been ensuring that these models make correct and meaningful inferences, especially in high-stakes decision-making scenarios. To address this, eXplainable AI (XAI) tools have been leveraged to shed light on the inner workings and decisions of ML models. However, explaining decisions made by sequential ML models—which make a series of decisions over time—remains complex and less understood. Existing XAI tools often fall short in offering clarity for such models, making it challenging for designers to evaluate and improve ML systems comprehensively. Hence, there is an acute need for more sophisticated and interactive XAI tools tailored to sequential ML models.
INNOVATION
Researchers in Professor Nikola Banovic's lab have created the Visual Interactive Model Explorer (VIME) which provides an advanced XAI toolbox that helps ML model designers explain and visualize decisions of sequential models. VIME enables users to explore various "what-if" scenarios, providing a more intuitive and detailed understanding of model behavior over time. This interactive approach allows for an easier identification and explanation of incorrect decisions by sequential ML models, as evidenced by an evaluation involving 14 ML experts. The evaluation demonstrated that VIME was significantly more effective than baseline XAI tools. With its capability to unravel the decision-making processes in a user-friendly, iterative manner, VIME has the potential for broad applications in domains like healthcare, finance, and autonomous systems where sequential decision-making is critical. By informing future interactive XAI designs, VIME paves the way for more reliable and transparent ML decision support systems.