Arrhythmia Classification Using Measurement of Cardiac Activity and Power Analysis
Technology Number: 2020-357
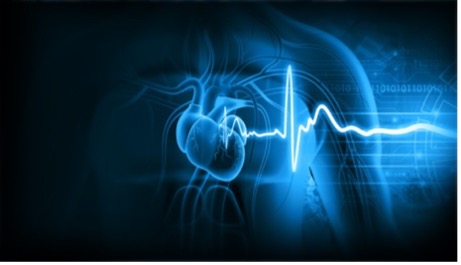
OVERVIEW
Arrhythmia classification system using advanced cardiac signal analysis
- Converts signals into multiple domains for comprehensive arrhythmia detection and classification
- Applies to detecting arrhythmias, classifying arrhythmia types, enhancing cardiac diagnostics
BACKGROUND
Understanding and diagnosing arrhythmias is critical for effective cardiac care. Traditional methods for arrhythmia detection often rely on electrocardiograms (ECGs), which can be limited in their ability to provide comprehensive data to accurately diagnose various types of arrhythmias. Historical approaches generally focus on single-domain analysis, capturing data in either the time or frequency domain, resulting in potential diagnostic inaccuracies and missed arrhythmic events. These methods also face challenges such as noise interference and limited signal quality. Furthermore, many systems lack the capability to classify different types of arrhythmias effectively, posing a significant challenge in clinical settings. This scenario underscores the need for an advanced and more reliable method for detecting and classifying arrhythmias to improve patient outcomes and assist clinicians in making more accurate diagnoses.
INNOVATION
The newly developed arrhythmia classification system addresses these shortcomings by collecting cardiac activity signals from any probing method and converting them into frequency, time, or phase domain representations for analysis. The evaluation stage extracts the energy density profile over various frequencies, time intervals, or phases, which is then summed and normalized to form dispersion metrics. These metrics are analyzed to determine whether a patient is experiencing an arrhythmia and to classify the type of arrhythmia. This multi-domain approach ensures a more comprehensive and accurate detection of arrhythmic events, reducing diagnostic errors. Real-world applications include early detection of arrhythmias, precise classification of different arrhythmic types, and improved cardiac diagnostics, ultimately enhancing patient care and treatment strategies.