Dictionary Learning for Improved Clinical Decision Making
TECHNOLOGY NUMBER: 2020-057
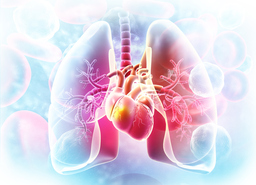
OVERVIEW
An automated machine method based on dictionary learning from CT images- Provides the ability to improve lung donor screening prior to transplantation
- May provide a means to increase the pool for acceptable donor lungs for transplantation
BACKGROUND
Lung donors are extremely rare, with strict criteria employed to select suitable donor lungs. The ideal lung donor has an age of <55 years of age, excellent blood gas parameters, short or no smoking history, and a clear chest radiograph. It is therefore not surprising that approximately 70% of evaluated lungs are declined for transplant. As donor lungs are therefore extremely rare, options are increasingly being explored to expand the donor pool, including the use of extended-criteria donors. If it were possible to find donors whose parameter fall below these ideal criteria while providing acceptable short- and long-term results, the pool of available lungs for transplantation would correspondingly expand.
INNOVATION
Researchers at the University of Michigan have developed an automated machine learning method based on dictionary learning of unique features extracted from medical images to improve lung donor screening prior to transplantation. The automated machine learning mechanism is based upon a dictionary approach to screen "good versus bad" lungs from the data provided by computed tomography scans. This distinction has been developed through the collection of 5,000,000 image patches of size 15 x 15 pixels from segmented lungs to train a dictionary for each class. The aim is to develop automated machine learning methods to help in screening donor lungs to determine if there is an association with transplanted lungs.